Achieving the right consistency - II
A statistical analysis of consistency among Test bowlers
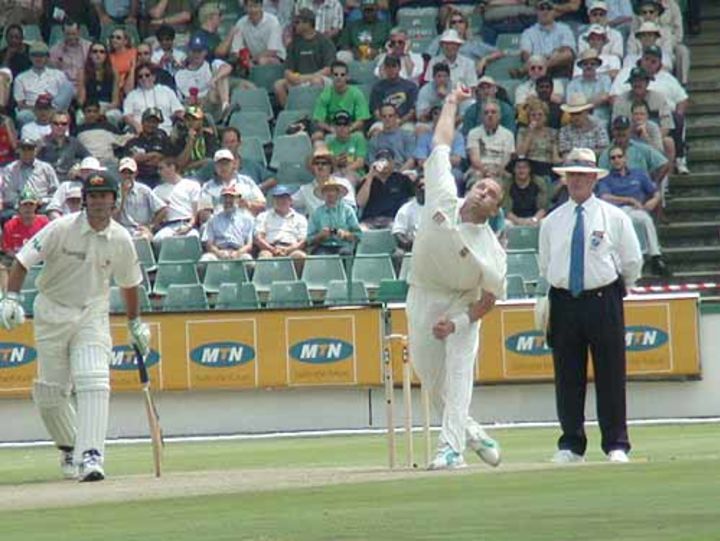
In my first column for It Figures, I took a look at innings-to-innings consistency among batsmen, and reached the conclusion that, on balance, it appears to be a good thing. This time around, I've performed an analysis looking at bowlers. My methods are identical, with particular reliance on the coefficient of variation (CoV) as an estimator of consistency; please see my previous post for full details.
At the outset, it should be noted that bowling stats present a small problem. Whereas our primary concern about batsmen is how many runs they score, we tend to be interested in two things with bowlers: how many wickets they take and how many runs they concede (and, of course, the standard measure by which we judge them – the bowling average – is a quotient of the two). The problem is that it is only straightforward to observe the innings-to-innings variability of one or other of these measures at a time. For the purposes of this analysis, then, I have just relied on wickets taken.
In a way, this is helpful: although it's not a stat on which we tend to focus much attention, wickets-per-innings (WPI) is the direct equivalent of runs-per-innings (or, give or take a little adjustment for not-outs, the batting average). It is also a good, sensible measure to use to think about bowling consistency: I hope most readers would agree that a bowler who takes 5/95, 5/176, and 5/23 in consecutive innings conforms more closely to our intuitive sense of bowling consistency than one who takes 1/30, 6/180, and 2/60, even though the latter took his wickets at an identical cost in each innings.
There are some fairly good reasons why WPI is a seldom-seen stat, however. The biggest problem is that it might be heavily influenced by factors over which the bowler has no control. You might be the finest bowler in your team but, unless your captain believes that, he won't ask you to bowl much and you won't take many wickets. Moreover, if the teammates with whom you share the ball are good bowlers, they are liable to take plenty of wickets, themselves, thereby depleting the finite number of scalps left for you to claim. (Pelham Barton has made the excellent point that batting in a team of good batsmen increases your opportunity to score runs, whereas bowling in a team of good bowlers reduces your opportunity to take wickets.) For these reasons, it might be argued that WPI tells us as much about the other players in a team as it reveals about the one in whom we're interested. This is fair enough: I have to acknowledge that a bowler might have a more or less consistent record for reasons for which he cannot, himself, take all the credit or blame, but that's a way to explain differences, rather than a rationale for assuming they don't exist.
Test consistency
There's a familiar name at the top of the most consistent bowlers list (Table 1). Unless something remarkable happens in his final game, Muttiah Muralitharan will retire not only as Test cricket's most prolific wicket-taker, but also as its most consistent. He has taken between 2 and 5 wickets in over two-thirds of the Test innings in which he has bowled, and his remaining analyses are fairly evenly divided between more and less successful returns. It is predictable that these characteristics would be reflected in an exceptionally low CoV.
Joel Garner may be an example of the type of bowler whose WPI is constrained by formidable competition for the scarce resource of opposition wickets. Seeing as he took at least 4 wickets in an innings 25 times, it's hard to imagine that he wouldn't have managed more than 7 fiver-fers if wickets hadn't invariably been tumbling at the other end, too.
In the upper reaches of a list that is dominated by some very high-class bowlers, Darren Gough's name may look a tiny bit out of place, but his low CoV is testament to his dependability at a time when his country's attack sorely needed it.
Name | M | I | W | Ave | W/I | SD | CoV | |
1. | M Muralitharan | 131 | 226 | 787 | 22.66 | 3.48 | 1.87 | 0.537 |
2. | CTB Turner | 17 | 30 | 101 | 16.53 | 3.37 | 1.89 | 0.561 |
3. | DW Steyn | 41 | 75 | 211 | 23.13 | 2.81 | 1.66 | 0.591 |
4. | WJ O'Reilly | 27 | 48 | 144 | 22.60 | 3.00 | 1.78 | 0.593 |
5. | R Peel | 20 | 35 | 101 | 16.98 | 2.89 | 1.75 | 0.607 |
6. | J Garner | 58 | 111 | 259 | 20.98 | 2.33 | 1.44 | 0.615 |
7. | CV Grimmett | 37 | 67 | 216 | 24.22 | 3.22 | 2.01 | 0.622 |
8. | D Gough | 58 | 95 | 229 | 28.40 | 2.41 | 1.53 | 0.633 |
9. | SF Barnes | 27 | 50 | 189 | 16.43 | 3.78 | 2.41 | 0.638 |
10. | AA Donald | 72 | 129 | 330 | 22.25 | 2.56 | 1.65 | 0.644 |
... | ||||||||
12. | DK Lillee | 70 | 132 | 355 | 23.92 | 2.69 | 1.79 | 0.665 |
... | ||||||||
15. | MD Marshall | 81 | 151 | 376 | 20.95 | 2.49 | 1.71 | 0.687 |
16. | B Lee | 75 | 148 | 308 | 30.71 | 2.08 | 1.44 | 0.690 |
... | ||||||||
19. | A Kumble | 132 | 236 | 619 | 29.65 | 2.62 | 1.84 | 0.700 |
20. | SK Warne | 144 | 271 | 702 | 25.53 | 2.59 | 1.82 | 0.701 |
21. | RJ Hadlee | 86 | 150 | 431 | 22.30 | 2.87 | 2.02 | 0.702 |
... | ||||||||
26. | FS Trueman | 67 | 127 | 307 | 21.58 | 2.42 | 1.78 | 0.737 |
... | ||||||||
30. | GD McGrath | 123 | 241 | 560 | 21.69 | 2.32 | 1.73 | 0.746 |
31. | SM Pollock | 108 | 202 | 421 | 23.12 | 2.08 | 1.56 | 0.747 |
... | ||||||||
33. | Wasim Akram | 104 | 181 | 414 | 23.62 | 2.29 | 1.73 | 0.754 |
... | ||||||||
38. | CEL Ambrose | 98 | 179 | 405 | 20.99 | 2.26 | 1.72 | 0.758 |
... | ||||||||
40. | Waqar Younis | 87 | 154 | 373 | 23.56 | 2.42 | 1.84 | 0.761 |
41. | Imran Khan | 88 | 142 | 362 | 22.81 | 2.55 | 1.94 | 0.763 |
42. | CA Walsh | 132 | 242 | 519 | 24.45 | 2.14 | 1.64 | 0.765 |
... | ||||||||
79. | IT Botham | 102 | 168 | 383 | 28.40 | 2.28 | 1.92 | 0.844 |
... | ||||||||
86. | GA Lohmann | 18 | 36 | 112 | 10.76 | 3.11 | 2.66 | 0.856 |
... | ||||||||
102. | JC Laker | 46 | 86 | 193 | 21.25 | 2.24 | 2.00 | 0.891 |
... | ||||||||
122. | Kapil Dev | 131 | 227 | 434 | 29.65 | 1.91 | 1.81 | 0.946 |
123. | DL Underwood | 86 | 151 | 297 | 25.84 | 1.97 | 1.86 | 0.946 |
... | ||||||||
125. | GS Sobers | 93 | 159 | 235 | 34.04 | 1.48 | 1.40 | 0.950 |
... | ||||||||
140. | JG Bracewell | 41 | 67 | 102 | 35.81 | 1.52 | 1.61 | 1.061 |
141. | JH Kallis | 139 | 230 | 265 | 31.57 | 1.15 | 1.23 | 1.067 |
142. | AW Greig | 58 | 93 | 141 | 32.21 | 1.52 | 1.62 | 1.071 |
143. | N Boje | 43 | 72 | 100 | 42.65 | 1.39 | 1.52 | 1.097 |
144. | RJ Shastri | 80 | 125 | 151 | 40.96 | 1.21 | 1.34 | 1.110 |
145. | MA Noble | 42 | 71 | 121 | 25.00 | 1.70 | 1.93 | 1.133 |
146. | R Illingworth | 61 | 100 | 122 | 31.20 | 1.22 | 1.43 | 1.168 |
147. | TE Bailey | 61 | 95 | 132 | 29.21 | 1.39 | 1.68 | 1.210 |
148. | W Rhodes | 58 | 90 | 127 | 26.97 | 1.41 | 1.81 | 1.285 |
149. | CL Hooper | 102 | 145 | 114 | 49.43 | 0.79 | 1.15 | 1.457 |
qual. 100 Test wickets; complete list available here |
I picked Derek Underwood out in the list because, of bowlers with any sort of reputation, he has one of the highest CoVs, indicating a less consistent innings-to-innings record. This comes about because there is a bit of a feast-or-famine profile to his Test wicket-taking. He took no more than one wicket in over half of the innings in which he bowled, but he also bagged 17 five-fers. Like the batsman who swings between cheap dismissals and big hundreds (remember Vinoo Mankad?), Deadly's record suggests that he could be inspirational or ineffectual in equal measure. His famous unplayability in particular conditions (above all, on drying wickets) might partly explain this finding.
In the main, the bowlers at the bottom of the list are allrounders and/or not especially penetrative spinners of the kind used to "tie up one end". This probably isn't a great surprise, since bowlers of these types are likely to take relatively few wickets in most of their innings (remember that, in these analyses, a bowler who takes 0, 0, 2, 1, & 0 wickets in consecutive innings is judged to be less consistent than one who takes 4, 4, 6, 5, & 4, even though the absolute variability within those hauls is identical). In addition, when these bowlers turn in a significant performance (as they all at least occasionally do), it stands out in much greater contrast from their typical level of achievement, and their SDs – and, consequently, CoVs – are increased. If a bowler with a WPI of 1 records a 5-wicket haul, that's 500% of his typical performance; for a bowler with a WPI of 2.5, the same feat would only be 200% of his norm.
The upshot is that there are a lot of good bowlers at the top of the list, and progressively fewer as consistency declines. As a result, it is no surprise to find a relatively pronounced correlation between CoV and bowling average (r 2=0.322; p<0.001). This relationship is illustrated in Figure 1; you can see that a substantial majority of bowlers who average under 30 have a CoV of less than 1.
Figure 2 shows the typical relationship between CoV and win-rate, with bowlers with the most consistent wicket-taking records apparently benefitting from a very slightly increased probability of victory, although the association is not an especially dramatic one (r 2=0.043; p<0.001).
There's an interesting twist in this story, though. You may recall that, when I looked at the same relationship for Test batsmen, I found that CoV was less strongly related to winning percentage than it was to not-losing percentage. This finding was explained by the fact that consistency is also associated with drawing rate (i.e. consistent batsmen are a bit more likely to win and a bit more likely to draw, with the net result that they're a fair bit less likely to lose). This phenomenon is not repeated amongst bowlers; in fact, something rather different is going on. The first thing I noticed was that, unlike their willow-wielding counterparts, more consistent bowlers are no less likely to lose matches (r 2<0.001; p=0.866). That, I thought, must mean there's something going on with drawn games that cancels out the benefit of consistency for winning. And that is exactly what proved to be the case: in a complete reversal of the situation for batsmen, the most consistent bowlers are less likely to draw Test matches (r 2=0.050; p<0.001). The only sensible way of explaining this, as far as I can see, is that consistent batsmen help their sides to draw matches they might otherwise have lost, whereas consistent bowlers help their sides to win matches they might otherwise have drawn.
ODI consistency
When I produced stats for the most consistent ODI wicket-takers, there were two unexpected names at the top of the list (Table 2).
I have to confess that I hadn't previously realised quite how good Chris Pringle's ODI bowling statistics are generally (for example, he amassed over 100 wickets at an average better than, say, Botham's or Marshall's or Imran's). What the current analysis emphasises is the way in which he achieved that record – namely, by chipping in dependably just about every time he played. His captains could invariably rely on him to contribute a dismissal or two (he went wicketless in just 10 of the 64 ODIs in which he bowled), though they couldn't really hope for many more (he only managed a four-fer or better on just three occasions). Similarly – in a career that has been anything but stable due to his terrible luck with injuries – Pringle's compatriot Kyle Mills has managed to piece together an admirably consistent wicket-taking record in ODIs. He has taken between 1 and 3 wickets in over three-quarters of his games. It is often said of New Zealand's ODI side of the last couple of decades that they have punched above their weight – that their players manage to play to something like their full ability in a reliable fashion, even if the fundamental level of that ability is perceived to be less than that seen in more glamorous teams. This analysis appears to provide a little support for that notion, if Pringle's and Mills's records are anything to go by (and Shane Bond isn't far behind).
Name | M | I | W | Ave | WPI | SD | CoV | |
1. | C Pringle | 64 | 64 | 103 | 23.87 | 1.61 | 1.15 | 0.717 |
2. | KD Mills | 108 | 109 | 162 | 26.46 | 1.49 | 1.11 | 0.744 |
3. | AA Donald | 164 | 162 | 272 | 21.79 | 1.68 | 1.26 | 0.748 |
4. | B Lee | 183 | 179 | 317 | 23.18 | 1.77 | 1.33 | 0.750 |
5. | CJ McDermott | 138 | 138 | 203 | 24.72 | 1.47 | 1.13 | 0.769 |
6. | SK Warne | 193 | 190 | 291 | 25.82 | 1.53 | 1.18 | 0.769 |
7. | MG Johnson | 81 | 80 | 128 | 25.72 | 1.60 | 1.23 | 0.769 |
8. | Saqlain Mushtaq | 169 | 165 | 288 | 21.79 | 1.75 | 1.36 | 0.779 |
9. | DW Fleming | 88 | 88 | 134 | 25.39 | 1.52 | 1.19 | 0.779 |
10. | DK Lillee | 63 | 63 | 103 | 20.83 | 1.63 | 1.28 | 0.781 |
... | ||||||||
12. | SE Bond | 78 | 80 | 147 | 20.88 | 1.84 | 1.45 | 0.791 |
... | ||||||||
15. | SCJ Broad | 65 | 65 | 109 | 25.76 | 1.68 | 1.34 | 0.797 |
... | ||||||||
19. | IT Botham | 116 | 115 | 145 | 28.54 | 1.26 | 1.01 | 0.804 |
20. | D Gough | 158 | 155 | 234 | 26.30 | 1.51 | 1.21 | 0.805 |
21. | M Muralitharan | 326 | 322 | 504 | 23.07 | 1.57 | 1.26 | 0.805 |
22. | GD McGrath | 245 | 245 | 377 | 22.06 | 1.54 | 1.25 | 0.812 |
... | ||||||||
26. | J Garner | 98 | 98 | 146 | 18.85 | 1.49 | 1.23 | 0.826 |
27. | Shoaib Akhtar | 142 | 141 | 221 | 23.64 | 1.57 | 1.31 | 0.833 |
... | ||||||||
32. | A Kumble | 269 | 263 | 334 | 30.84 | 1.27 | 1.09 | 0.855 |
33. | A Flintoff | 138 | 116 | 168 | 23.62 | 1.45 | 1.24 | 0.857 |
... | ||||||||
36. | Kapil Dev | 225 | 221 | 253 | 27.45 | 1.14 | 1.00 | 0.870 |
... | ||||||||
44. | Wasim Akram | 356 | 351 | 502 | 23.53 | 1.43 | 1.27 | 0.885 |
... | ||||||||
47. | Waqar Younis | 262 | 258 | 416 | 23.84 | 1.61 | 1.43 | 0.890 |
... | ||||||||
49. | RJ Hadlee | 115 | 112 | 158 | 21.56 | 1.41 | 1.26 | 0.891 |
50. | SM Pollock | 294 | 291 | 387 | 24.31 | 1.33 | 1.19 | 0.893 |
... | ||||||||
52. | Harbhajan Singh | 209 | 201 | 238 | 32.97 | 1.18 | 1.06 | 0.896 |
... | ||||||||
56. | Imran Khan | 175 | 153 | 182 | 26.62 | 1.19 | 1.11 | 0.936 |
57. | CEL Ambrose | 176 | 175 | 225 | 24.13 | 1.29 | 1.21 | 0.940 |
58. | WPUJC Vaas | 321 | 319 | 399 | 27.46 | 1.25 | 1.18 | 0.945 |
... | ||||||||
60. | MD Marshall | 136 | 134 | 157 | 26.96 | 1.17 | 1.11 | 0.947 |
... | ||||||||
85. | JH Kallis | 298 | 261 | 250 | 32.12 | 0.96 | 1.04 | 1.090 |
... | ||||||||
93. | ST Jayasuriya | 440 | 364 | 319 | 36.65 | 0.88 | 1.10 | 1.257 |
94. | IVA Richards | 187 | 131 | 118 | 35.83 | 0.90 | 1.16 | 1.286 |
95. | SB Styris | 165 | 144 | 125 | 34.95 | 0.87 | 1.12 | 1.290 |
96. | WJ Cronje | 188 | 153 | 114 | 34.79 | 0.75 | 0.97 | 1.307 |
97. | Azhar Mahmood | 142 | 139 | 123 | 39.13 | 0.88 | 1.17 | 1.322 |
98. | PA de Silva | 308 | 156 | 106 | 39.41 | 0.68 | 0.90 | 1.323 |
99. | GW Flower | 219 | 154 | 104 | 40.26 | 0.68 | 0.95 | 1.411 |
100. | PD Collingwood | 182 | 139 | 103 | 38.86 | 0.74 | 1.05 | 1.414 |
101. | SR Tendulkar | 442 | 267 | 154 | 44.27 | 0.58 | 0.95 | 1.641 |
102. | SC Ganguly | 308 | 170 | 100 | 38.35 | 0.59 | 1.00 | 1.706 |
qual. 100 ODI wickets; complete list available here |
Again, the lower reaches of the table are dominated by bits-and-pieces players, who might provide occasional match-altering spells (even Ganguly and Tendulkar each have two ODI five-fers under their belts), but are more commonly asked to use up overs (17 of the bottom 20 contribute fewer than one wicket per ODI).
Gough – along with three Australian heroes in the assorted shapes of Dennis Lillee, Shane Warne, and Brett Lee – makes the top 20s of both lists, but there's one player who ranks among the 10 most consistent for both Tests and ODIs, and that's Allan Donald. His skipper could reliably expect over 2½ wickets per test innings and 1⅔ scalps per ODI from him. Maybe that doesn't sound like much, but it's equivalent to saying that – match-in, match-out – Donald could be depended upon to contribute his share of opposition wickets, and probably somewhat more, whenever he took the ball (and regardless of whether that ball was red or white).
There's a very pronounced correlation between CoV and ODI bowling average (r 2=0.557; p<0.001), with increasing inconsistency obviously reflected in increasing averages (Figure 3). This association is a bit stronger than we saw in Test bowling figures. One possible explanation is that, because the amount of bowling available to any one bowler is constrained in ODIs (usually to 10 overs), WPI becomes a purer measure of a bowler's contribution, and consistency in this measure becomes a more direct index of out-and-out quality with the ball. One way or another, though, it's very clear that better ODI bowlers tend to be more consistent ODI bowlers.
The relationship between bowling consistency and ODI win-rate (Figure 4) is not quite as obvious, though it certainly appears that bowlers with lower CoVs tend to be more likely to win their games (r 2=0.034; p=0.007).
Conclusions
To at least as marked a degree as we saw with batsmen, consistent bowlers appear to be worth having on your team. And it can't be a surprise to learn that the bowlers who take wickets most dependably tend to be those with the lowest averages.
However, we have to be careful with our conclusions, this time. It may be that good bowlers with low averages are asked to bowl more, so they end up taking wickets more consistently. Perhaps less good bowlers would take wickets with equal consistency – though not as high frequency – given the opportunity. In this way, the fact that allrounders and certain types of spin bowlers have the least consistent WPI records may reflect the ways in which they are used as much as it reveals anything fundamental about the bowlers themselves (this takes us back to general concerns about the meaningfulness of WPI as a stat). For instance, we can assume that, if he batted like Chris Martin, Jacques Kallis would have been asked to take a greater share of South Africa's bowling, over the years. Had that been the case, he would surely have taken more wickets, and he would probably also have taken wickets more consistently. His record would not have been so dominated by 0- and 1-wicket innings (69% of his Tests and 72% of his ODIs fall into this category), and his significant wicket-taking bursts would have been much less occasional. As a result, we would expect him to climb the consistency table – perhaps markedly so. But, just because we think we can explain Kallis's relatively inconsistent record, that doesn't mean that his record is, in some way, actually more consistent than we're giving him credit for.
The relationship between consistency and winning is similar for bowlers as we saw for batsmen (although the finding that consistent bowlers draw fewer Test matches is an interesting one). Again, I tend to conclude that, in both forms of the game, teams benefit from dependable – though not necessarily dazzling – contributors at least as much as they do from hit-or-miss performers. However, the overall range of variation is somewhat narrower amongst bowlers: it appears that, while there really is such a thing as a ton-or-bust batsman, bowlers who are capable of significant hauls but commonly contribute little are altogether rarer beasts.
All stats calculated Jul 04, 2010 (i.e. all Tests up to West Indies v South Africa at Bridgetown, Jun 26-29, 2010 [Test # 1962] and all ODIs up to England v Australia at Lord's, Jul 3, 2010 [ODI # 3011]).
Technical appendix
(Some notes on my methods, for anyone who's almost as dull as me. Everyone else can stop reading now.)
Technical note #1. All regressions are limited to bowlers with at least 50 wickets. I'd have preferred to use fuller datasets, but they're just too noisy (probably due to the phenomenon of the truly occasional bowler).
Technical note #2. As last time, I performed a multivariate regression on these data. For both forms of the game, I regressed CoV against average, winning percentage, and an interaction term. In each instance, the only significant covariate was average (p<0.001). This suggests that the reason more consistent bowlers win more Test matches and ODIs is that they average less: there is no independent effect of consistency on winning.
Technical note #3.I said in my introduction that there is no straightforward way of measuring variability in wickets taken and runs conceded simultaneously. I can think of quite complicated ways, though. One approach would be to use a Poisson regression model, with wickets as events considered against an exposure variable of runs conceded. Such an analysis is a little beyond the scope of this kind of column, and I have some reservations about the strict applicability of the paradigm. Nevertheless, if anyone's remotely interested, I might try to find a moment to do explore this sort of approach.
Read in App
Elevate your reading experience on ESPNcricinfo App.