Consistency in Test bowlers: a new look
An improved way of analysing consistency across the career of Test bowlers statistically
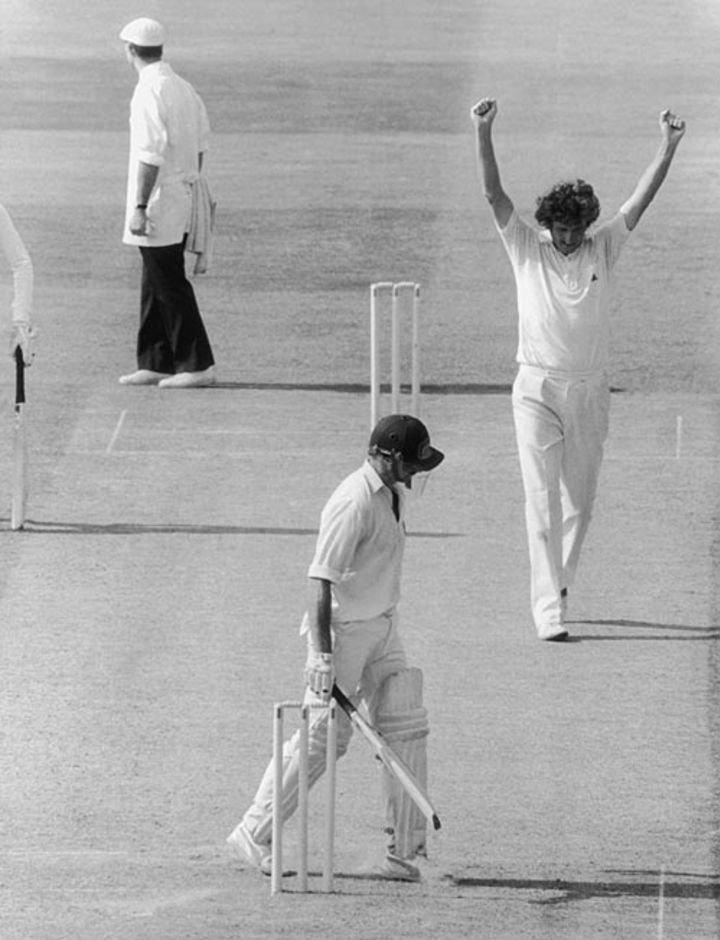
This is based on an idea given by Prashanth. After giving the idea and participating in a discussion or two, he disappeared off the radar. However I thank him for providing the spark. Couple of years back Gabriel Rogers did a similar article. However that wonderful article was based on complex statistical methodology and would not have been out of place in an Annual Conference of Statisticians. Mine is simpler, more common-sense based and is aimed at everyone who comes into this blogspace, irrespective of his statistical knowledge.
The relevant points are explained below.
1. For this purposefive-Test slicesare considered. This is a reasonable number and normally covers 2-3 months of Test cricket. Tests, rather than innings are used as the basis so that both bowling and batting can be covered in an equitable manner.
2. Five tests means that batsman can go through a Test or two of limited opportunities to bat or non-batting because of emphatic wins etc. There will be enough opportunities within the five-Test slice to catch up. Normally the bowlers do not have this problem since they do a higher share of a team's work and have to capture 20 wickets for a win.
3. There is enough time to get over short duration loss of form.
4. To measure consistency,only runs scored and wickets captured will be used. The fundamental cricket dictum that batsmen should score runs and bowlers should take wickets is followed. Averages are important mainly over a career and for comparisons across players.
5. Why not average? Let us take couple of examples to understand why not. McGrath and Trueman have career averages around 22.0 and WpT values of around 4.5. In a 5-Test period, match context being comparable, McGrath captures 25 wickets at 25.0 and Trueman, 15 wickets at 20.0. Who has performed closer to his career figures and for that matter, better. Certainly McGrath, despite the higher slice average. Similarly for batting.
6. Let us not forget that we remember numbers like 46 (Laker) and 41 (Alderman) rather than the specific averages. Similarly 774 (Gavaskar) and 688 (Lara) without being aware of the averages.
7. The career slices should be non-overlapping and equal, other than the last one. Gooch's 456 in one test should be part of one career slice only. Similarly Laker's 19 wickets. Hence the concept of rolling number of Tests is not valid.
8. Five Tests might seem arbitrary but represents a long enough career slice. It represents a long Test series.
9. The keyword isconsistency with reference to the player's own career performance levels. It may happen that a bowler has a rather high WpT value: e-g, Barnes at 7.00, Muralitharan at 6.02 et al., and what is perfectly acceptable for another bowler might not be, for such bowlers. That is acceptable since they have set high benchmarks and we are interested in seeing how often they went off these benchmarks.
10. We are not looking about high and low values but only relative to the concerned player's career figures. Over a five-Test stretch Murali is expected to take 30 wickets and Kallis is expected to capture nine wickets. This will be the basis. If Murali captured 20 wickets in the Test slice, it is well below average and the same 20-wickets performance for Kallis, way above average.
11. I know that a bowler like Imran Khan who did not bowl at all in 3 slices at the end of his career would be slightly affected by this methodology. However there is no clear method of handling this. I do not want to exclude Tests where a bowler did not bowl. Then the number of slices would not be dependent on the number of Tests played. Also I don't want someone later on asking me to exclude batsman's Test where there has been an innings win for loss of few wickets. These are minor quirks and may only reduce the accuracy from 100% to 95%.
12. Adjustment is made for the last career slice if the same is fewer than five Tests.
13. The criteria for selection is 100 or more Test wickets. 160 bowlers qualify. The only bowlers of note who are missing are Shane Bond and Frank Tyson (Adrian, happy !!!).
14.TheStandard Deviation (SD)of the slice ratios is used to determine consistency.
I had initially thought that I would combine the batsmen and bowlers together in a single article. However the introduction of six tall graphs meant that the article would have become very long and I have separated this into two articles. The graphs are also special purpose ones showing the slice plotting of up to 10 players per graph.
The following 5 groups are formed for purposes of determining consistency. For each career-slice of 5-tests, a ratio is formed between that concerned slice's runs/wickets and the career-average runs/wickets for 5 tests. This ratio is calledSPF (Slice Performance Factor). Suppose the bowler has captured 17 wickets and his 5-Test career-WpT value is 24, the SPF value is 0.71. If he captured 30 wickets, the SPF is 1.25.
A. SPF below 0.67: Well below average - Falls into the inconsistent bracket. B. SPF 0.67 - 0.90: Below average C. SPF 0.90 - 1.10: Around average D. SPF 1.10 - 1.33: Above average E. SPF above 1.33: Well above average - Falls into the inconsistent bracket.
Groups B, C and D are considered to be well within the average levels. Standard Deviation is also used to determine the consistency.
First some data tables. The first one is the core table of bowlers who have captured over 300 wickets in their Test career. The tables and graphs are presented with least comments. Let me allow the erudite readers to come out with their own comments.
Bowler | Tests | Wkts | Avge | WpT | Mean | StdDev | Mid3% | C-Slices | Grp A | Grp B | Grp C | Grp D | Grp E |
---|---|---|---|---|---|---|---|---|---|---|---|---|---|
Muralitharan M | 133 | 800 | 22.73 | 6.0 | 1.00 | 0.274 | 74.1 | 27 | 4 | 5 | 10 | 5 | 3 |
Warne S.K | 145 | 708 | 25.42 | 4.9 | 1.00 | 0.295 | 69.0 | 29 | 4 | 6 | 9 | 5 | 5 |
Kumble A | 132 | 619 | 29.65 | 4.7 | 1.00 | 0.275 | 74.1 | 27 | 3 | 7 | 5 | 8 | 4 |
McGrath G.D | 124 | 563 | 21.64 | 4.5 | 1.00 | 0.266 | 72.0 | 25 | 3 | 5 | 8 | 5 | 4 |
Walsh C.A | 132 | 519 | 24.44 | 3.9 | 1.01 | 0.328 | 70.4 | 27 | 3 | 8 | 7 | 4 | 5 |
Kapil Dev N | 131 | 434 | 29.65 | 3.3 | 0.99 | 0.390 | 48.1 | 27 | 7 | 7 | 3 | 3 | 7 |
Hadlee R.J | 86 | 431 | 22.30 | 5.0 | 1.03 | 0.283 | 83.3 | 18 | 1 | 3 | 10 | 2 | 2 |
Pollock S.M | 108 | 421 | 23.12 | 3.9 | 1.00 | 0.274 | 72.7 | 22 | 2 | 7 | 5 | 4 | 4 |
Wasim Akram | 104 | 414 | 23.62 | 4.0 | 0.99 | 0.337 | 66.7 | 21 | 4 | 3 | 6 | 5 | 3 |
Harbhajan Singh | 98 | 406 | 32.22 | 4.1 | 0.99 | 0.261 | 85.0 | 20 | 3 | 3 | 6 | 8 | 0 |
Ambrose C.E.L | 98 | 405 | 20.99 | 4.1 | 1.00 | 0.287 | 70.0 | 20 | 2 | 8 | 4 | 2 | 4 |
Ntini M | 101 | 390 | 28.83 | 3.9 | 1.00 | 0.355 | 61.9 | 21 | 2 | 8 | 2 | 3 | 6 |
Botham I.T | 102 | 383 | 28.40 | 3.8 | 1.01 | 0.429 | 52.4 | 21 | 6 | 4 | 2 | 5 | 4 |
Marshall M.D | 81 | 376 | 20.95 | 4.6 | 0.99 | 0.292 | 70.6 | 17 | 3 | 3 | 4 | 5 | 2 |
Waqar Younis | 87 | 373 | 23.56 | 4.3 | 1.00 | 0.430 | 55.6 | 18 | 4 | 7 | 1 | 2 | 4 |
Imran Khan | 88 | 362 | 22.81 | 4.1 | 0.98 | 0.464 | 55.6 | 18 | 5 | 1 | 4 | 5 | 3 |
Vettori D.L | 110 | 358 | 33.87 | 3.3 | 1.00 | 0.410 | 68.2 | 22 | 4 | 6 | 3 | 6 | 3 |
Lillee D.K | 70 | 355 | 23.92 | 5.1 | 1.00 | 0.243 | 71.4 | 14 | 2 | 2 | 6 | 2 | 2 |
Vaas WPUJC | 111 | 355 | 29.58 | 3.2 | 1.00 | 0.376 | 69.6 | 23 | 4 | 6 | 4 | 6 | 3 |
Donald A.A | 72 | 330 | 22.25 | 4.6 | 1.00 | 0.226 | 86.7 | 15 | 1 | 5 | 4 | 4 | 1 |
Willis R.G.D | 90 | 325 | 25.20 | 3.6 | 1.00 | 0.174 | 94.4 | 18 | 1 | 4 | 6 | 7 | 0 |
Lee B | 76 | 310 | 30.82 | 4.1 | 1.00 | 0.266 | 87.5 | 16 | 0 | 9 | 2 | 3 | 2 |
Gibbs L.R | 79 | 309 | 29.09 | 3.9 | 0.99 | 0.275 | 68.8 | 16 | 3 | 3 | 4 | 4 | 2 |
Trueman F.S | 67 | 307 | 21.58 | 4.6 | 0.99 | 0.300 | 71.4 | 14 | 2 | 4 | 5 | 1 | 2 |
To clarify the table contents. WpT mean Wickets per test. Mean is the mean of the SPF values and is close to 1.0 for all bowlers. StdDev is the Standard Deviation for all the SPF values. Mid3% is the % of the Groups B, C and D over the total number of Career Slices, which is the next column: C-Slices. Grp A to Grp E are self-explanatory. The complete file is available for downloading. The link is provided at the end.
Amongst the top wicket-takers, only Hadlee and Harbhajan Singh have the Mid3% values exceeding 80, indicating a high level of consistency. Then comes Donald, with 86% and Willis, with a very high 94%.
Consistency is determined in two ways. The first is statistical. The Standard Deviation (SD) is determined for all the ratios. Low SD values indicate consistent players and high SD values indicate inconsistent players. The usual method of using the Coefficient of Variation is not required since the means for almost all players is around 1.00. Shown below are the SD tables with the low-20 SDs indicating very consistent bowlers.
Bowler | Tests | Wkts | Avge | WpT | Mean | StdDev | Mid3% | C-Slices | Grp A | Grp B | Grp C | Grp D | Grp E |
---|---|---|---|---|---|---|---|---|---|---|---|---|---|
O'Reilly W.J | 27 | 144 | 22.60 | 5.3 | 1.00 | 0.120 | 100.0 | 6 | 0 | 1 | 4 | 1 | 0 |
Morkel M | 39 | 139 | 30.04 | 3.6 | 1.00 | 0.152 | 100.0 | 8 | 0 | 2 | 4 | 2 | 0 |
Adcock N.A.T | 26 | 104 | 21.11 | 4.0 | 1.00 | 0.158 | 100.0 | 6 | 0 | 2 | 2 | 2 | 0 |
Dilley G.R | 41 | 138 | 29.76 | 3.4 | 0.98 | 0.169 | 100.0 | 9 | 0 | 3 | 4 | 2 | 0 |
Kasprowicz M.S | 38 | 113 | 32.88 | 3.0 | 0.99 | 0.171 | 87.5 | 8 | 0 | 2 | 4 | 1 | 1 |
Willis R.G.D | 90 | 325 | 25.20 | 3.6 | 1.00 | 0.174 | 94.4 | 18 | 1 | 4 | 6 | 7 | 0 |
Snow J.A | 49 | 202 | 26.67 | 4.1 | 1.00 | 0.194 | 90.0 | 10 | 0 | 3 | 5 | 1 | 1 |
Collinge R.O | 35 | 116 | 29.25 | 3.3 | 1.00 | 0.196 | 85.7 | 7 | 1 | 1 | 2 | 3 | 0 |
Lohmann G.A | 18 | 112 | 10.76 | 6.2 | 1.02 | 0.200 | 100.0 | 4 | 0 | 1 | 1 | 2 | 0 |
Old C.M | 46 | 143 | 28.11 | 3.1 | 1.02 | 0.202 | 100.0 | 10 | 0 | 3 | 3 | 4 | 0 |
Saeed Ajmal | 20 | 107 | 26.70 | 5.3 | 1.00 | 0.210 | 100.0 | 4 | 0 | 1 | 1 | 2 | 0 |
Danish Kaneria | 61 | 261 | 34.80 | 4.3 | 0.99 | 0.211 | 84.6 | 13 | 1 | 3 | 6 | 2 | 1 |
Umar Gul | 43 | 157 | 32.48 | 3.7 | 1.00 | 0.219 | 88.9 | 9 | 0 | 3 | 3 | 2 | 1 |
Johnson I.W | 45 | 109 | 29.19 | 2.4 | 1.00 | 0.222 | 77.8 | 9 | 1 | 1 | 4 | 2 | 1 |
Steyn D.W | 54 | 272 | 23.19 | 5.0 | 0.99 | 0.223 | 81.8 | 11 | 1 | 2 | 5 | 2 | 1 |
Hughes M.G | 53 | 212 | 28.38 | 4.0 | 0.99 | 0.224 | 90.9 | 11 | 1 | 3 | 1 | 6 | 0 |
Donald A.A | 72 | 330 | 22.25 | 4.6 | 1.00 | 0.226 | 86.7 | 15 | 1 | 5 | 4 | 4 | 1 |
Statham J.B | 70 | 252 | 24.85 | 3.6 | 1.00 | 0.230 | 78.6 | 14 | 2 | 2 | 6 | 3 | 1 |
Johnson M.G | 47 | 190 | 31.29 | 4.0 | 1.00 | 0.236 | 70.0 | 10 | 1 | 2 | 5 | 0 | 2 |
Edmonds P.H | 51 | 125 | 34.18 | 2.5 | 1.00 | 0.239 | 81.8 | 11 | 1 | 3 | 4 | 2 | 1 |
Now for the tables with the high-SD values indicating a very low level of inconsistency.
Bowler | Tests | Wkts | Avge | WpT | Mean | StdDev | Mid3% | C-Slices | Grp A | Grp B | Grp C | Grp D | Grp E |
---|---|---|---|---|---|---|---|---|---|---|---|---|---|
Rhodes W | 58 | 127 | 26.97 | 2.2 | 1.00 | 0.887 | 33.3 | 12 | 5 | 1 | 2 | 1 | 3 |
Hooper C.L | 102 | 114 | 49.43 | 1.1 | 1.01 | 0.727 | 23.8 | 21 | 9 | 4 | 0 | 1 | 7 |
Briggs J | 33 | 118 | 17.75 | 3.6 | 0.97 | 0.679 | 57.1 | 7 | 2 | 1 | 2 | 1 | 1 |
Hogg R.M | 38 | 123 | 28.45 | 3.2 | 0.99 | 0.569 | 75.0 | 8 | 1 | 5 | 1 | 0 | 1 |
Bracewell J.G | 41 | 102 | 35.81 | 2.5 | 1.13 | 0.563 | 55.6 | 9 | 2 | 2 | 1 | 2 | 2 |
Illingworth R | 61 | 122 | 31.20 | 2.0 | 0.99 | 0.555 | 61.5 | 13 | 3 | 5 | 2 | 1 | 2 |
Kallis J.H | 152 | 276 | 32.45 | 1.8 | 1.00 | 0.547 | 38.7 | 31 | 10 | 6 | 2 | 4 | 9 |
Sobers G.St.A | 93 | 235 | 34.04 | 2.5 | 1.00 | 0.529 | 52.6 | 19 | 4 | 4 | 2 | 4 | 5 |
Giffen G | 31 | 103 | 27.10 | 3.3 | 0.98 | 0.523 | 42.9 | 7 | 2 | 1 | 2 | 0 | 2 |
Shastri R.J | 80 | 151 | 40.96 | 1.9 | 1.00 | 0.512 | 62.5 | 16 | 3 | 6 | 3 | 1 | 3 |
Verity H | 40 | 144 | 24.38 | 3.6 | 1.00 | 0.511 | 62.5 | 8 | 2 | 1 | 3 | 1 | 1 |
Noble M.A | 42 | 121 | 25.00 | 2.9 | 1.02 | 0.508 | 55.6 | 9 | 2 | 3 | 1 | 1 | 2 |
Underwood D.L | 86 | 297 | 25.84 | 3.5 | 1.06 | 0.496 | 33.3 | 18 | 6 | 2 | 2 | 2 | 6 |
Mushtaq Ahmed | 52 | 185 | 32.97 | 3.6 | 1.00 | 0.485 | 54.5 | 11 | 2 | 4 | 1 | 1 | 3 |
Intikhab Alam | 47 | 125 | 35.95 | 2.7 | 1.01 | 0.481 | 30.0 | 10 | 3 | 1 | 1 | 1 | 4 |
Greig A.W | 58 | 141 | 32.21 | 2.4 | 0.99 | 0.479 | 58.3 | 12 | 3 | 3 | 4 | 0 | 2 |
Giles A.F | 54 | 143 | 40.60 | 2.6 | 0.99 | 0.473 | 45.5 | 11 | 3 | 2 | 3 | 0 | 3 |
Abdul Qadir | 67 | 236 | 32.81 | 3.5 | 1.00 | 0.465 | 50.0 | 14 | 3 | 3 | 3 | 1 | 4 |
Imran Khan | 88 | 362 | 22.81 | 4.1 | 0.98 | 0.464 | 55.6 | 18 | 5 | 1 | 4 | 5 | 3 |
Bailey T.E | 61 | 132 | 29.21 | 2.2 | 1.00 | 0.463 | 30.8 | 13 | 6 | 2 | 0 | 2 | 3 |
The alternate method is common-sense-based. The two extreme group numbers, A and E, are considered significant departures from the career levels. The middle three group numbers are added and divided by the total number of slices to get the Mid3%. This reflects the consistency of the players. Shown below are the SD tables with the high-10 Mid3% values.
Bowler | Tests | Wkts | Avge | WpT | Mean | StdDev | Mid3% | C-Slices | Grp A | Grp B | Grp C | Grp D | Grp E |
---|---|---|---|---|---|---|---|---|---|---|---|---|---|
O'Reilly W.J | 27 | 144 | 22.60 | 5.3 | 1.00 | 0.120 | 100.0 | 6 | 0 | 1 | 4 | 1 | 0 |
Old C.M | 46 | 143 | 28.11 | 3.1 | 1.02 | 0.202 | 100.0 | 10 | 0 | 3 | 3 | 4 | 0 |
Morkel M | 39 | 139 | 30.04 | 3.6 | 1.00 | 0.152 | 100.0 | 8 | 0 | 2 | 4 | 2 | 0 |
Dilley G.R | 41 | 138 | 29.76 | 3.4 | 0.98 | 0.169 | 100.0 | 9 | 0 | 3 | 4 | 2 | 0 |
Lohmann G.A | 18 | 112 | 10.76 | 6.2 | 1.02 | 0.200 | 100.0 | 4 | 0 | 1 | 1 | 2 | 0 |
Saeed Ajmal | 20 | 107 | 26.70 | 5.3 | 1.00 | 0.210 | 100.0 | 4 | 0 | 1 | 1 | 2 | 0 |
Adcock N.A.T | 26 | 104 | 21.11 | 4.0 | 1.00 | 0.158 | 100.0 | 6 | 0 | 2 | 2 | 2 | 0 |
Willis R.G.D | 90 | 325 | 25.20 | 3.6 | 1.00 | 0.174 | 94.4 | 18 | 1 | 4 | 6 | 7 | 0 |
Hughes M.G | 53 | 212 | 28.38 | 4.0 | 0.99 | 0.224 | 90.9 | 11 | 1 | 3 | 1 | 6 | 0 |
Snow J.A | 49 | 202 | 26.67 | 4.1 | 1.00 | 0.194 | 90.0 | 10 | 0 | 3 | 5 | 1 | 1 |
Now for the tables with the low Mid3% values indicating a very low level of inconsistency.
Bowler | Tests | Wkts | Avge | WpT | Mean | StdDev | Mid3% | C-Slices | Grp A | Grp B | Grp C | Grp D | Grp E |
---|---|---|---|---|---|---|---|---|---|---|---|---|---|
Hooper C.L | 102 | 114 | 49.43 | 1.1 | 1.01 | 0.727 | 23.8 | 21 | 9 | 4 | 0 | 1 | 7 |
Intikhab Alam | 47 | 125 | 35.95 | 2.7 | 1.01 | 0.481 | 30.0 | 10 | 3 | 1 | 1 | 1 | 4 |
Bailey T.E | 61 | 132 | 29.21 | 2.2 | 1.00 | 0.463 | 30.8 | 13 | 6 | 2 | 0 | 2 | 3 |
Underwood D.L | 86 | 297 | 25.84 | 3.5 | 1.06 | 0.496 | 33.3 | 18 | 6 | 2 | 2 | 2 | 6 |
Rhodes W | 58 | 127 | 26.97 | 2.2 | 1.00 | 0.887 | 33.3 | 12 | 5 | 1 | 2 | 1 | 3 |
Pathan I.K | 29 | 100 | 32.26 | 3.4 | 0.99 | 0.363 | 33.3 | 6 | 2 | 0 | 2 | 0 | 2 |
Boje N | 43 | 100 | 42.65 | 2.3 | 0.99 | 0.429 | 33.3 | 9 | 3 | 2 | 1 | 0 | 3 |
Benaud R | 63 | 248 | 27.03 | 3.9 | 0.99 | 0.442 | 38.5 | 13 | 4 | 3 | 0 | 2 | 4 |
Kallis J.H | 152 | 276 | 32.45 | 1.8 | 1.00 | 0.547 | 38.7 | 31 | 10 | 6 | 2 | 4 | 9 |
Yadav N.S | 35 | 102 | 35.10 | 2.9 | 1.00 | 0.407 | 42.9 | 7 | 2 | 3 | 0 | 0 | 2 |
Not surprisingly there is a strong negative correlation between the two methods. Understandably the correlation is negative since low SD and high Mid3% values indicate consistency. The correlation coefficient is a fairly high -0.73.
Now for some special graphs.
© Anantha Narayanan
The top-10 bowlers are featured. It can be clearly seen that most of these bowlers do not exhibit a high level of consistency. The only exception seems to be Hadlee, during the first half of his career.
Most consistent: Based on low SD values
© Anantha Narayanan
Look at O'Reilly. An SD a low as 0.12 indicates a very consistent career. This is borne out by his placement in the next graph also. Willis is the only one amongst this lot with over 85 Tests. The others have all played below 50 Tests. Amongst the modern bowlers, Kasprowicz and Steyn have been fairly consistent. Especially look at Steyn's last five slices.
Most consistent: Based on high Middle-3-group % values
© Anantha Narayanan
These are the bowlers with high middle three group % values. There are four bowlers, led by O'Reilly who have all their groups in the middle. This is amazing. This means that not once did these bowlers go below 66.7% or above 133.3% of their career values. That is some consistency. It can be seen that three of these bowlers, O'Reilly, Dilley and Adcock also occupy the top three positions in the SD table, indicating the very high degree of correlation between the two methods. Old is there in the top-10. However in terms of consistency, Willis takes the plum position. Look at his graph. Out of 18 career slices only once has he gone into the two extreme groups.
Least consistent: Based on high SD values
© Anantha Narayanan
These graphs look like the dying person's cardiograph. These players have had moves up and down throughout their career. Most of them are also batting all-rounders. It is also possible that these players might have had stretches in which they bowled very little. However that means that they were very inconsistent as bowlers.
Least consistent: Based on low Middle-3-group % values
© Anantha Narayanan
Almost the same bowlers. However now Underwood and Benaud come in. Look at Pathan's graph. Most of these bowlers have around a third of the slices in the middle.
Bowlers with top averages
© Anantha Narayanan
Just to complete the analysis I have given here the charts for the top bowlers - by average., since most of them would have missed the first chart: by wickets captured. Again inconsistency seems to be the trend here. But look at Adcock. High consistency, coupled with low average. And look at Barnes's chart. One nice long position just under the mean, made up at the end. Marshall and Ambrose seem to have alternating low and highs. Note Laker's huge spike, obviously during 1956.
I think mention must be made of two bowlers, Bill O'Reilly and Bob Willis. O'Reilly never went off the middle three groups. That is some consistency. Willis, for someone who played 85 Tests, went off the middle radar just once. That is again the definition of consistency.
To download/view the Excel sheet containing the complete data for 160 bowlers, please click/right-click here.
I will do a similar analysis for Batsmen next.