Extreme batting - fastest and slowest innings in Tests
What are the fastest and slowest Test innings of all time
Charles Davis
25-Feb-2013
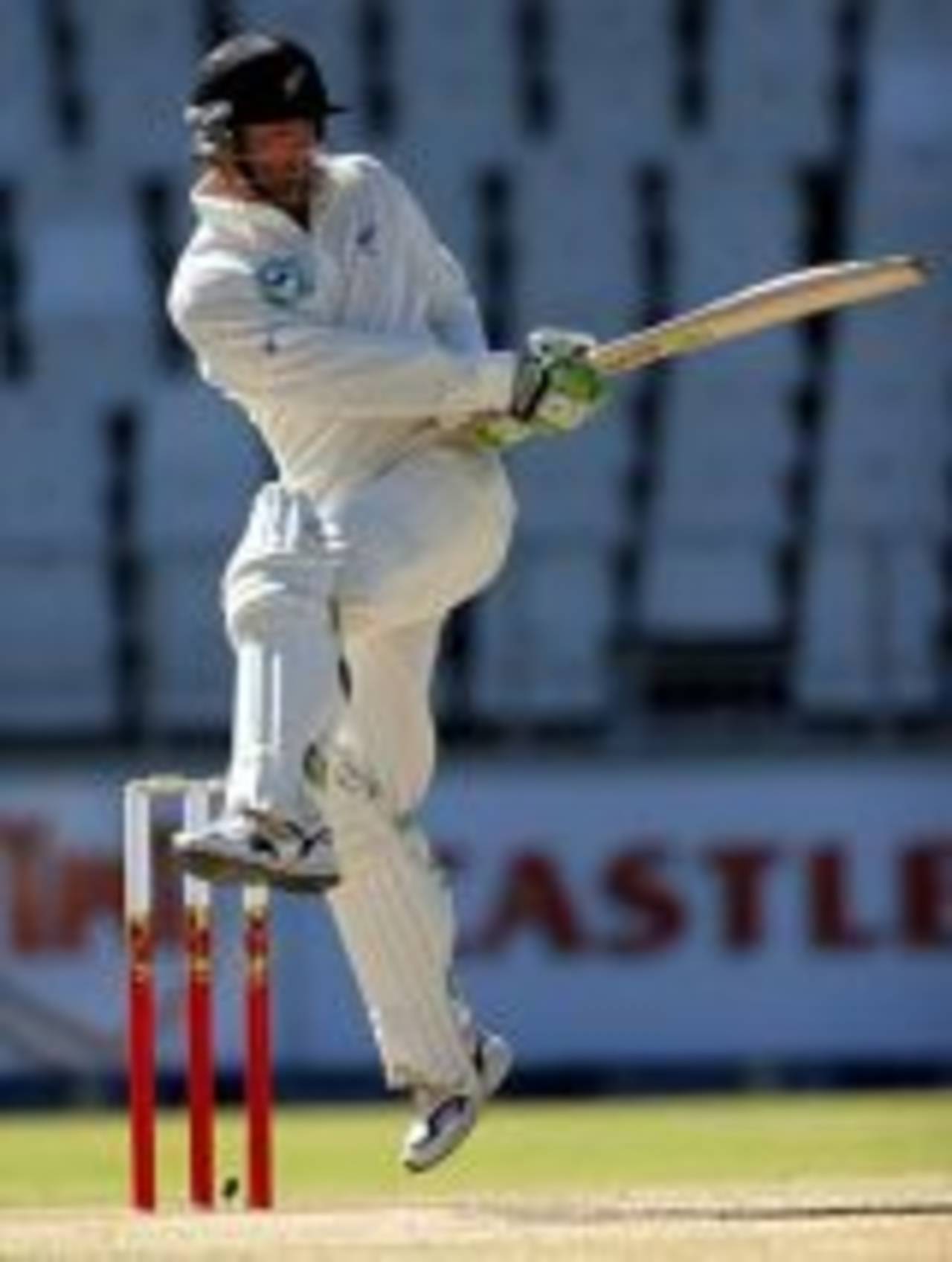
Neil Lane/ESPNcricinfo Ltd
What are the fastest and slowest Test innings of all time?
A simple question like this is actually tricky, thanks to the extreme range of possible scores. Comparing innings large and small, based on scoring speed alone, is unsatisfactory. For instance, Adam Gilchrist’s 102 off 59 balls in 2006 was considerably faster that Nathan Astle’s 222 off 168 balls in 2002; both were freakish innings, but which was the more remarkable?
One way to answer this is by measuring how far each innings deviates from normal innings of similar size. To do this, we take every innings of a given size – in terms of runs scored – calculate the average (or mean) balls faced, and then calculate the standard deviation, which is a measure of the spread or variability of the data. We can then give the most exceptional innings a z-score (the number of standard deviations from the mean) which becomes a measure of how extraordinary the innings were.
An example may help clarify this. Let’s look at all innings of exactly 76 runs in Test matches. We have balls faced data for 119 such innings. The average number of balls faced is 161 and the standard deviation of this data is about 49.
The fastest known innings of 76 in Tests was off 72 balls by Viv Richards in Adelaide in 1980. This is 1.75 standard deviations faster than the average, so the innings gets a z-score of -1.75. Likewise, the slowest innings of 76 was 315 balls by Glenn Turner in 1971, with a z-score of +3.2.
To compare many innings of different sizes, the process must be repeated for all possible scores. This process gives big innings a better rating than smaller innings of a similar speed, because it is more difficult to score rapidly for longer periods.
So which innings have the most extreme z-scores? At fast end of the scale, the results look like this:
Batsman | Runs | Balls | Match | Venue & year | z-score |
---|---|---|---|---|---|
Viv Richards | 110 | 58 | WI v Eng | Antigua, 1986 | -2.58 |
Nathan Astle | 222 | 168 | NZ v Eng | Christchurch, 2002 | -2.54 |
Adam Gilchrist | 102 | 59 | Aus v Eng | Perth, 2006 | -2.45 |
Chris Cairns | 82 | 47 | NZ v Eng | Lord’s, 2004 | -2.36 |
Jack Gregory | 119 | 81 | Aus v SA | Johannesburg, 1921 | -2.34 |
Jacques Kallis | 54 | 25 | SA v Zim | Cape Town, 2005 | -2.34 |
Kapil Dev | 89 | 55 | Ind v Eng | Lord’s, 1982 | -2.33 |
Recent innings are prominent in this list, a sign of the speed of the modern game. Still, no batsman has reached quite the extremes of Viv Richards in his record-breaking century in 1986. I wonder what it is about English bowling that has attracted so many extreme innings.
At the other end of the scale, we must go further back in time.
Batsman | Runs | Balls | Match | Venue & year | z-score |
---|---|---|---|---|---|
Hanif Mohammad | 20 | 223 | Pak v Eng | Lord’s, 1954 | 7.90 |
Alec Bannerman | 91 | 620 | Aus v Eng | Sydney, 1892 | 7.88 |
Herbie Collins | 40 | 340 | Aus v Eng | Manchester, 1921 | 7.66 |
John Murray | 3 | 100 | Eng v Aus | Sydney, 1963 | 7.15 |
Yashpal Sharma | 13 | 159 | Ind v Aus | Adelaide, 1981 | 6.90 |
Geoff Allott | 0 | 77 | NZ v SA | Auckland, 1999 | 6.80 |
It is interesting to see a wide range of scores, from 0 to 91, appearing on this list. Modern cricket watchers can only wonder at the extremes represented here. In terms of time, Hanif would have, going by modern-day over-rates, taken more than five hours for his 20 runs, while Alec Bannerman’s 91 would probably take more than two full days. Apart from Bannerman, every other batsman who has faced 620 or more balls in a Test innings has scored well over 200 runs, and the most balls faced (known) in reaching a century is 525 by Colin Cowdrey in 1957. Perhaps it is no wonder that Bannerman, unlike his more adventurous brother Charles, never scored a Test century.
Of course, there are quite a number of past innings for which balls faced are unknown, so we don’t know exactly where they may fit on the scale, but we can still make some estimates. Of particular interest is Dilip Sardesai’s 60 against the West Indies in Bridgetown in 1962. Sardesai was at the crease for 155 overs, and probably faced over 450 balls; if so, his z-score would be 7.93. His dismissal in that match started an extraordinary collapse that saw Lance Gibbs take eight wickets for six runs.
A postscript puzzle: innings of four runs, on average, involve fewer balls faced than innings of three runs. There is a logical reason for this (for readers to ponder).
[Notes for the statistically-minded: this process works quite well when we have data available for a very large number of innings. However, it does require some smoothing and trend-fitting at higher, rarer scores (above 120). Note also that the distributions are skewed, so z-scores of fast innings are different in magnitude to slow ones, and at the fast end of the scale the calculation is not very useful for innings of less than 40 runs. However, the process is still useful as long as we just compare fast with fast, and slow with slow.]