The vexed question of 'not outs' in Test cricket
The statistical measurement of a player's batting average is one that has survived unaltered for 130 years of Test cricket - but it suffers from a fundamental flaw in the way 'not outs' are handled
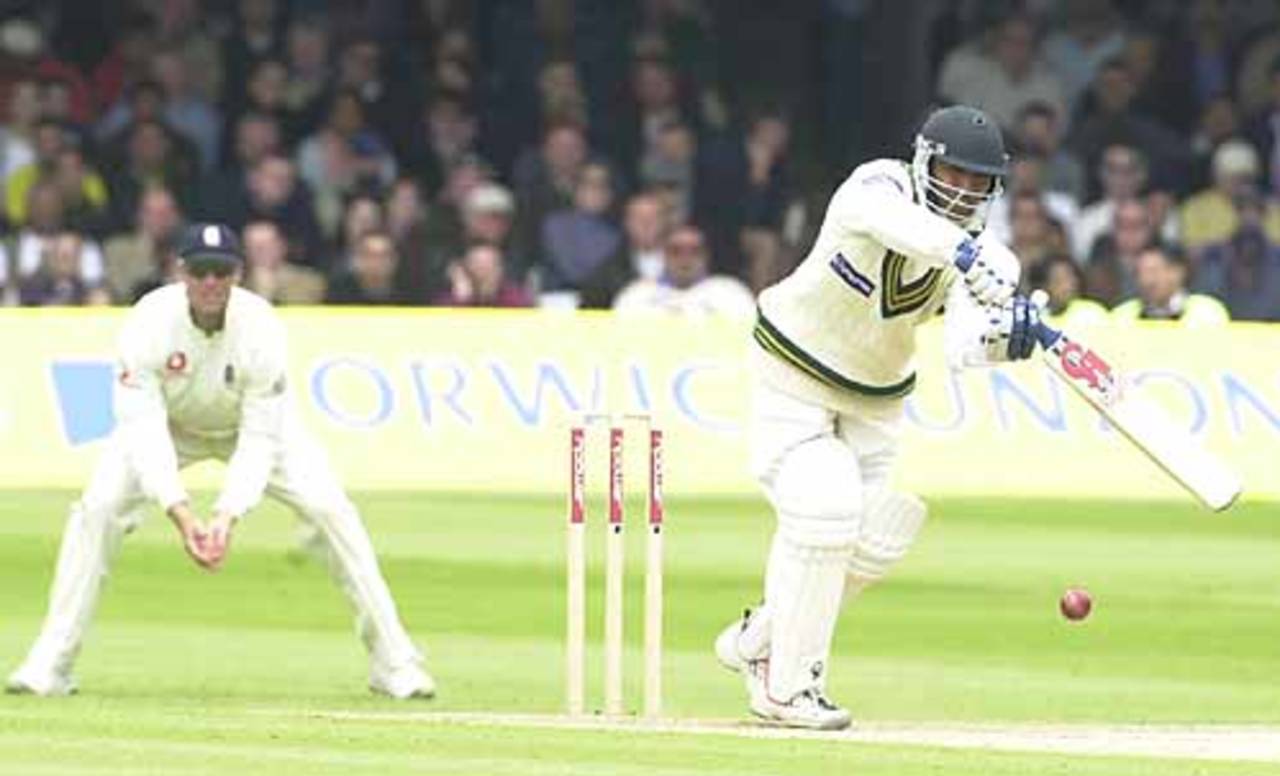
Among batsmen with 4000-plus runs, Saeed Anwar is the only one to have an Extended Batting average greater than his Test average • Paul McGregor/ESPNcricinfo Ltd
So what exactly is the problem? Well, it lies in the manner of handling not outs. Lara played an epic, scoring 400 runs over 13 hours but this innings, as far as determining the batting average is concerned, does not exist. On the other hand, his three first-ball ducks against Australia, England and New Zealand are considered as three innings. While it is true that he was dismissed in the later three innings, it is also a fact that he played long enough to have played four complete innings. Basically 'batting average' should not exclude such innings.
As Milind puts it quite effectively, the batting average computation violates a basic mathematical dictum. Runs are added to the numerator and nothing to the denominator. Absolutely perfect description of the anomaly that exists.
Let us compare the figures of two modern great batsmen.
Batsman Team T I No SNo No % Runs Avge RpI Kallis J.H Saf 162 274 40 5 14.6 13128 56.10 47.91 Lara B.C Win 131 232 6 2 2.6 11953 52.89 51.52
Kallis has played 31 more Tests to score additional 1150 runs but averages just over three runs more. That is because Kallis has 40 not outs compared with Lara's four. It might be due to the way Lara played, his batting positions or more declarations for Kallis who is a part of a stronger team and so on. Let us see how we can address the anomaly which is somewhat unfair to the top-order batsmen.
It should be noted that this problem is more pronounced in ODI matches because of the limited number of overs available and absence of declarations. It is also a fact that two batsmen remain not out in most ODI innings. However ODI batting is measured by the batting average and strike-rate, thus lowering the singular importance of batting averages.
I have selected 34 batsmen, who have scored over 2000 Test runs and averaged over 50, for this analysis. Virender Sehwag is just hanging on by the skin of his teeth and a failure in Chennai may very well plunge him below 50. And a reasonable Test at Centurion would push de Villiers past the 50 mark. However the data for all batsmen who have crossed 2000 runs is available for downloading and the link is provided later. The data is current up to match 2073, the Cape Town Test which finished just now.
Batsman | Team | Tests | Inns | No | No % | Runs | Avge |
---|---|---|---|---|---|---|---|
Bradman D.G | Aus | 52 | 80 | 10 | 12.5 | 6996 | 99.94 |
Pollock R.G | Saf | 23 | 41 | 4 | 9.8 | 2256 | 60.97 |
Headley G.A | Win | 22 | 40 | 4 | 10.0 | 2190 | 60.83 |
Sutcliffe H | Eng | 54 | 84 | 9 | 10.7 | 4555 | 60.73 |
Barrington | Eng | 82 | 131 | 15 | 11.5 | 6806 | 58.67 |
EdeC Weekes | Win | 48 | 81 | 5 | 6.2 | 4455 | 58.62 |
Hammond W.R | Eng | 85 | 140 | 16 | 11.4 | 7249 | 58.46 |
Sobers | Win | 93 | 160 | 21 | 13.1 | 8032 | 57.78 |
Hobbs J.B | Eng | 61 | 102 | 7 | 6.9 | 5410 | 56.95 |
Walcott C.L | Win | 44 | 74 | 7 | 9.5 | 3798 | 56.69 |
Hutton L | Eng | 79 | 138 | 15 | 10.9 | 6971 | 56.67 |
Kallis J.H | Saf | 162 | 274 | 40 | 14.6 | 13128 | 56.10 |
Sangakkara | Slk | 115 | 196 | 16 | 8.2 | 10045 | 55.81 |
Tendulkar | Ind | 194 | 320 | 32 | 10.0 | 15645 | 54.32 |
Chappell | Aus | 87 | 151 | 19 | 12.6 | 7110 | 53.86 |
Nourse A.D | Saf | 34 | 62 | 7 | 11.3 | 2960 | 53.82 |
Lara B.C | Win | 131 | 232 | 6 | 2.6 | 11953 | 52.89 |
Miandad | Pak | 124 | 189 | 21 | 11.1 | 8832 | 52.57 |
Clarke M.J | Aus | 89 | 148 | 15 | 10.1 | 6989 | 52.55 |
Dravid R | Ind | 164 | 286 | 32 | 11.2 | 13288 | 52.31 |
Mohd Yousuf | Pak | 90 | 156 | 12 | 7.7 | 7530 | 52.29 |
Amla H.M | Saf | 68 | 118 | 10 | 8.5 | 5610 | 51.94 |
Ponting R.T | Aus | 168 | 287 | 29 | 10.1 | 13378 | 51.85 |
Chanderpaul | Win | 146 | 249 | 42 | 16.9 | 10696 | 51.67 |
Flower A | Zim | 63 | 112 | 19 | 17.0 | 4794 | 51.55 |
Hussey | Aus | 79 | 137 | 16 | 11.7 | 6235 | 51.53 |
Gavaskar | Ind | 125 | 214 | 16 | 7.5 | 10122 | 51.12 |
Waugh S.R | Aus | 168 | 260 | 46 | 17.7 | 10927 | 51.06 |
Younis Khan | Pak | 80 | 140 | 11 | 7.9 | 6580 | 51.01 |
Hayden M.L | Aus | 103 | 184 | 14 | 7.6 | 8626 | 50.74 |
Border A.R | Aus | 156 | 265 | 44 | 16.6 | 11174 | 50.56 |
Richards | Win | 121 | 182 | 12 | 6.6 | 8540 | 50.24 |
Compton | Eng | 78 | 131 | 15 | 11.5 | 5807 | 50.06 |
Sehwag V | Ind | 102 | 177 | 6 | 3.4 | 8559 | 50.05 |
Most cricket followers are au fait with the above table. The one data element not shown normally is the "Not out %". This shows the % of not outs out of the total innings played. Among this elite collection of 34 batsmen, who account for 13% of runs scored in Test cricket, the highest % of not outs has been achieved by Steve Waugh, the middle-order giant from Australia. He has been unbeaten one in six innings. Andy Flower, Shivnarine Chanderpaul and Allan Border have similar numbers. In Flower's case, it has been more a question of a top drawer batsman in a weak team remaining unbeaten as his compatriots were dismissed.
The lowest figure has been achieved by Lara with 2.6%: that means once in 40 innings. Sehwag, with his attacking instincts is the only other batsman who clocks in fewer than 5%.
Out of interest, let me share with the readers some facts related to not outs across the 135 years of Test cricket. Of the 72865 innings played, there have been 9502 not outs, accounting for about 13%. Out of these 9502, 4253 not outs - nearly half - have been at scores below 10 runs.
A simple alternative is to use the Runs per Innings (RpI) instead of the batting average. Unfortunately it is a drastic step taking the other extreme. It affects the middle-order batsmen considerably. Many of their low-score not outs would be considered as completed innings and players like Kallis would be penalised. The graph below illustrates the two extreme situations - batting averages and RpI.
We need something between Batting average and RpI. I am proposing two alternatives to fill this space.
The first method seeks to redefine the not out innings. A dismissal is a dismissal and nothing needs to be done about those. But let us accept that even an Icelander with scant knowledge of cricket would accept that a 13-hour innings should not suddenly cease to exist just because of a declaration. Let us classify not out innings as "real not out" innings and the "Completed (or fulfilled) not out" innings.
The key is to determine a cut-off point beyond which the innings is considered as completed or fulfilled. I considered various values. A fixed figure, say, 25 or 50, would be unfair to weaker batsmen with low averages which means the figure has to be dynamically determined. The batting average itself is a good cut-off but a little stiff. Also we are questioning the very methodology of batting average. So I have zeroed in on a sensible dynamic value - a cut-off point at 50% of the "Average for dismissed innings". Here are couple of examples. Don Bradman's average for dismissed innings is 83.83 and any not out innings below 42 will be considered as a "real not out". Ken Barrington's average for dismissed innings is 50.37 and any not out innings below 25 will be considered as a "real not out". Any other not out innings would be considered as a fulfilled innings.
Let us examine the impact of this method. The table below lists the same 34 batsmen with their RpI and RpFI values, ordered by RpFI.
Batsman | Team | Tests | Inns | No | FulfilNO | Runs | Avge | RpI | RpFI | Chg % |
---|---|---|---|---|---|---|---|---|---|---|
Bradman D.G | Aus | 52 | 80 | 10 | 2 | 6996 | 99.94 | 87.45 | 89.69 | 10.3% |
Headley G.A | Win | 22 | 40 | 4 | 1 | 2190 | 60.83 | 54.75 | 56.15 | 7.7% |
EdeC Weekes | Win | 48 | 81 | 5 | 1 | 4455 | 58.62 | 55.00 | 55.69 | 5.0% |
Sutcliffe H | Eng | 54 | 84 | 9 | 2 | 4555 | 60.73 | 54.23 | 55.55 | 8.5% |
Hobbs J.B | Eng | 61 | 102 | 7 | 4 | 5410 | 56.95 | 53.04 | 55.20 | 3.1% |
Pollock R.G | Saf | 23 | 41 | 4 | 0 | 2256 | 60.97 | 55.02 | 55.02 | 9.8% |
Barrington | Eng | 82 | 131 | 15 | 4 | 6806 | 58.67 | 51.95 | 53.59 | 8.7% |
Walcott C.L | Win | 44 | 74 | 7 | 3 | 3798 | 56.69 | 51.32 | 53.49 | 5.6% |
Hammond W.R | Eng | 85 | 140 | 16 | 3 | 7249 | 58.46 | 51.78 | 52.91 | 9.5% |
Sangakkara | Slk | 115 | 196 | 16 | 3 | 10045 | 55.81 | 51.25 | 52.05 | 6.7% |
Hutton L | Eng | 79 | 138 | 15 | 4 | 6971 | 56.67 | 50.51 | 52.02 | 8.2% |
Lara B.C | Win | 131 | 232 | 6 | 2 | 11953 | 52.89 | 51.52 | 51.97 | 1.7% |
Sobers | Win | 93 | 160 | 21 | 4 | 8032 | 57.78 | 50.20 | 51.49 | 10.9% |
Tendulkar | Ind | 194 | 320 | 32 | 8 | 15645 | 54.32 | 48.89 | 50.14 | 7.7% |
Chappell | Aus | 87 | 151 | 19 | 8 | 7110 | 53.86 | 47.09 | 49.72 | 7.7% |
Nourse A.D | Saf | 34 | 62 | 7 | 2 | 2960 | 53.82 | 47.74 | 49.33 | 8.3% |
Mohd Yousuf | Pak | 90 | 156 | 12 | 3 | 7530 | 52.29 | 48.27 | 49.22 | 5.9% |
Sehwag V | Ind | 102 | 177 | 6 | 3 | 8559 | 50.05 | 48.36 | 49.19 | 1.7% |
Kallis J.H | Saf | 162 | 274 | 40 | 5 | 13128 | 56.10 | 47.91 | 48.80 | 13.0% |
Hayden M.L | Aus | 103 | 184 | 14 | 8 | 8626 | 50.74 | 46.88 | 49.01 | 3.4% |
Gavaskar | Ind | 125 | 214 | 16 | 4 | 10122 | 51.12 | 47.30 | 48.20 | 5.7% |
Younis Khan | Pak | 80 | 140 | 11 | 3 | 6580 | 51.01 | 47.00 | 48.03 | 5.8% |
Clarke M.J | Aus | 89 | 148 | 15 | 2 | 6989 | 52.55 | 47.22 | 47.87 | 8.9% |
Dravid R | Ind | 164 | 286 | 32 | 8 | 13288 | 52.31 | 46.46 | 47.80 | 8.6% |
Miandad | Pak | 124 | 189 | 21 | 4 | 8832 | 52.57 | 46.73 | 47.74 | 9.2% |
Richards | Win | 121 | 182 | 12 | 3 | 8540 | 50.24 | 46.92 | 47.71 | 5.0% |
Ponting R.T | Aus | 168 | 287 | 29 | 6 | 13378 | 51.85 | 46.61 | 47.61 | 8.2% |
Amla H.M | Saf | 68 | 118 | 10 | 0 | 5610 | 51.94 | 47.54 | 47.54 | 8.5% |
Hussey | Aus | 79 | 137 | 16 | 2 | 6235 | 51.53 | 45.51 | 46.19 | 10.4% |
Compton | Eng | 78 | 131 | 15 | 5 | 5807 | 50.06 | 44.33 | 46.09 | 7.9% |
Flower A | Zim | 63 | 112 | 19 | 5 | 4794 | 51.55 | 42.80 | 44.80 | 13.1% |
Chanderpaul | Win | 146 | 249 | 42 | 4 | 10696 | 51.67 | 42.96 | 43.66 | 15.5% |
Waugh S.R | Aus | 168 | 260 | 46 | 9 | 10927 | 51.06 | 42.03 | 43.53 | 14.7% |
Border A.R | Aus | 156 | 265 | 44 | 5 | 11174 | 50.56 | 42.17 | 42.98 | 15.0% |
It is obvious that the RpFI figures for batsmen with a high % of not outs would be much below the Batting average than those with low % of not outs. Bradman drops 10.3% & Kallis drops by 13.1%. Readers can note that the four middle-order batsmen who have already been discussed earlier possessing high % of not outs, viz., Andy Flower, Chanderpaul, Steve Waugh and Border have had the highest drops and occupy the bottom four positions in this table. The lowest drop has been for Lara and Sehwag, with 1.7%. In fact Sehwag, who was 34th in the batting average table moves up to 18th here. Even the high batting average of Kallis drops to below 50.
This is a simple and easy-to-understand method. Anyone can incorporate these figures by inspecting the not out innings of a batsman. I also have to accept that while this addresses the "not out" problem somewhat, the fundamental weakness of having an innings represented in the numerator in the form of runs and being ignored in the denominator exists. Albeit small innings only. At least the 400s and 365s have been taken care of.
However a more intuitive and stronger method is the one that tackles the "Runs" side of the formula to equate every batsman on a fair basis. In this method I will "extend" the not out innings to its natural conclusion or in other words - get the batsmen "out". Clearly this is a case of an extrapolation combining actual runs scored with virtual ones. Does it matter? Let us venture outside the normal realm of things and scrutinise what is in store.
The key question is "by how many runs" to extend these not out innings. When I started working on this idea a few years back, along with Dr.Ashwin Mahesh, we picked out the Batting average. In view of our own fundamental objection to this value we moved on to the RpI and subsequently to the "Average for dismissed innings". This is relatively easy to handle. Just multiply the number of not out innings by the "Average for dismissed innings", get the new total runs and divide by the total innings to derive the Extended Batting average (EBA). This can be added on to any existing table in a jiffy.
A few years back I noticed a flaw in this approach. Sehwag is batting these days like a village team slogger who has forgotten the basics. If, by any chance, he remains not out, however much it is unlikely, should we add nearly 50 runs to his innings? With all due respects to the great Tendulkar, a similar situation exists in his case too. That brings us to Michael Clarke and his purple patch. In the last 10 innings he has averaged over 80. It would be unfair to add only 45 runs or thereabouts.
Hence I decided that, despite the risk of adding complexity, I would add the Runs per innings for the last 10 innings played by the batsman. This is complex since this value has to be determined dynamically for each and every not out innings played by the batsman during his career. It requires tricky computer algorithms. Also note that I have used Runs per innings because we are considering only 10 innings and a couple of not out innings would distort the entire process. Why 10 innings instead of 10 Test matches? Well there have been times when a player played 3-5 Tests a year and it would have taken a few years to play 10 Tests. That is too long a period for a recent form connotation. In general, 10 innings is one long or two short series and would reflect the recent form quite accurately.
Let us peruse the revised figures. The table below lists the same 34 batsmen ordered by EBAvge.
Batsman | Team | Tests | Inns | Runs | Avge | OutAvge | ExtRuns | EBAvge | Chg % |
---|---|---|---|---|---|---|---|---|---|
Bradman D.G | Aus | 52 | 80 | 6996 | 99.94 | 83.83 | 7759 | 96.99 | 3.0% |
Sutcliffe H | Eng | 54 | 84 | 4555 | 60.73 | 54.64 | 5024 | 59.81 | 1.5% |
Pollock R.G | Saf | 23 | 41 | 2256 | 60.97 | 54.43 | 2394 | 58.39 | 4.2% |
EdeC Weekes | Win | 48 | 81 | 4455 | 58.62 | 54.88 | 4654 | 57.46 | 2.0% |
Hammond W.R | Eng | 85 | 140 | 7249 | 58.46 | 46.19 | 8018 | 57.27 | 2.0% |
Headley G.A | Win | 22 | 40 | 2190 | 60.83 | 45.61 | 2275 | 56.88 | 6.5% |
Barrington | Eng | 82 | 131 | 6806 | 58.67 | 50.37 | 7410 | 56.56 | 3.6% |
Hobbs J.B | Eng | 61 | 102 | 5410 | 56.95 | 53.34 | 5645 | 55.34 | 2.8% |
Hutton L | Eng | 79 | 138 | 6971 | 56.67 | 47.89 | 7629 | 55.28 | 2.5% |
Sangakkara | Slk | 115 | 196 | 10045 | 55.81 | 47.56 | 10792 | 55.06 | 2.3% |
Sobers | Win | 93 | 160 | 8032 | 57.78 | 44.06 | 8768 | 54.80 | 5.2% |
Kallis J.H | Saf | 162 | 274 | 13128 | 56.10 | 42.23 | 14905 | 54.40 | 3.0% |
Walcott C.L | Win | 44 | 74 | 3798 | 56.69 | 51.03 | 4001 | 54.07 | 4.6% |
Tendulkar | Ind | 194 | 320 | 15645 | 54.32 | 44.56 | 16888 | 52.77 | 2.8% |
Lara B.C | Win | 131 | 232 | 11953 | 52.89 | 49.76 | 12220 | 52.67 | 0.4% |
Mohd Yousuf | Pak | 90 | 156 | 7530 | 52.29 | 46.19 | 8009 | 51.34 | 1.8% |
Amla H.M | Saf | 68 | 118 | 5610 | 51.94 | 39.92 | 6042 | 51.20 | 1.4% |
Nourse A.D | Saf | 34 | 62 | 2960 | 53.82 | 47.49 | 3167 | 51.08 | 5.1% |
Clarke M.J | Aus | 89 | 148 | 6989 | 52.55 | 42.23 | 7559 | 51.07 | 2.8% |
Chappell | Aus | 87 | 151 | 7110 | 53.86 | 44.57 | 7706 | 51.03 | 5.3% |
Ponting R.T | Aus | 168 | 287 | 13378 | 51.85 | 45.15 | 14646 | 51.03 | 1.6% |
Dravid R | Ind | 164 | 286 | 13288 | 52.31 | 44.71 | 14505 | 50.72 | 3.1% |
Hayden M.L | Aus | 103 | 184 | 8626 | 50.74 | 47.68 | 9244 | 50.24 | 1.0% |
Sehwag V | Ind | 102 | 177 | 8559 | 50.05 | 47.96 | 8854 | 50.02 | 0.1% |
Younis Khan | Pak | 80 | 140 | 6580 | 51.01 | 44.24 | 6966 | 49.76 | 2.5% |
Miandad | Pak | 124 | 189 | 8832 | 52.57 | 41.97 | 9310 | 49.26 | 6.3% |
Chanderpaul | Win | 146 | 249 | 10696 | 51.67 | 34.49 | 12259 | 49.23 | 4.7% |
Hussey | Aus | 79 | 137 | 6235 | 51.53 | 42.50 | 6742 | 49.21 | 4.5% |
Gavaskar | Ind | 125 | 214 | 10122 | 51.12 | 44.14 | 10523 | 49.17 | 3.8% |
Compton | Eng | 78 | 131 | 5807 | 50.06 | 44.40 | 6302 | 48.11 | 3.9% |
Richards | Win | 121 | 182 | 8540 | 50.24 | 44.49 | 8753 | 48.09 | 4.3% |
Waugh S.R | Aus | 168 | 260 | 10927 | 51.06 | 35.47 | 12480 | 48.00 | 6.0% |
Flower A | Zim | 63 | 112 | 4794 | 51.55 | 35.43 | 5337 | 47.65 | 7.6% |
Border A.R | Aus | 156 | 265 | 11174 | 50.56 | 37.04 | 12397 | 46.78 | 7.5% |
Bradman's EBA is 97% of his Batting average, a drop of 3%. Headley drops 6.5%. Sobers drops by 5%. All the middle order stalwarts have drops exceeding 6%. Sehwag has the lowest drop: only 0.1%, virtually no change. Similarly Lara drops by only 0.4%. Amongst these top batsmen not even a single batsman has his EBA higher than his Batting average. This happens lower down the table. Mohsin Khan has the highest increase: 1.4%. The much-maligned Graeme Hick's EBA is 1.3% higher than his Batting average. Darren Ganga follows next with a 0.9% increase. A total of 11 batsmen have higher EBA values. Interested readers can study the Excel sheet for details. Saeed Anwar is the only batsman with more than 4000 runs under his belt and an EBA higher than Batting average.
This method is more elegant and intuitive with complexity of calculations being the sole deterrent. However the concept is very good and any cricket follower can implement the fixed value concept easily. The fixed value can be anything from a slew of values. And we can say with certainty that every innings is represented in the numerator and denominator. We have addressed that problem effectively.
Let us revisit the figures of Kallis and Lara.
Batsman Team T I No SNo No % Runs Avge RpI RpAI ExtRuns EBA %Avge Kallis J.H Saf 162 274 40 5 14.6 13128 56.10 47.91 48.80 14905 54.40 97.0% Lara B.C Win 131 232 6 2 2.6 11953 52.89 51.52 51.97 12220 52.67 99.6%Readers can see that Lara's average was nearly 4 fewer than Kallis. However his RpI and RpAI are nearly 3 runs higher. Significantly, the EBA, which is more accurate and a valid measure, is only less than 2 runs below Kallis. EBA probably reflects the central tendency most accurately.
Now for a revised graph. The two alternatives are pictorially represented occupying the space in the middle.
This is not a theoretical exercise - Two alternatives are presented to address a genuine problem. The Spl Not outs method is simple and easy to implement. The Extended Batting average method is more complex and would require a computer to incorporate recent form. However using the Out Bat average or Batting average or RpI or RpFI as the extension basis would be easier to implement. What is needed? Well, an influential organization such as ESPNcricinfo should study the suggestions and start implementing the revised averages: Of course along with the current measures.
To download/view the comprehensive Excel sheet containing the values for all the 264 batsmen who have crossed 2000 Test runs, please CLICK HERE.
Anantha Narayanan has written for ESPNcricinfo and CastrolCricket and worked with a number of companies on their cricket performance ratings-related systems