Kohli, Smith or Root: who's the most consistent of them all?
We look at how evenly (or not) a batsman's good scores are spread across their career
Himanish Ganjoo
08-Feb-2021
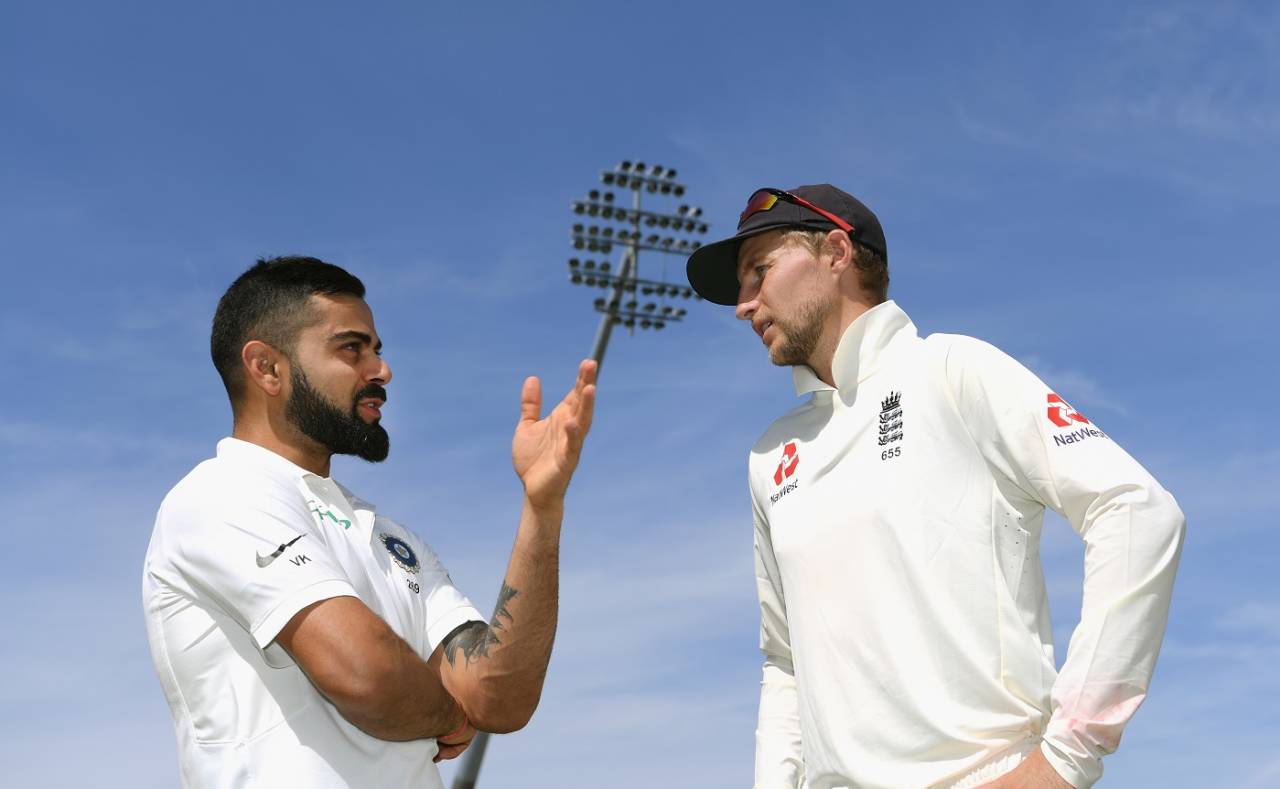
Kohli's Test career features extreme highs broken by lean phases, while Root is quite a contrast • Getty Images
Don Bradman's most frequent score is a duck.
You read that right. The man who averaged nearly a hundred runs per dismissal got out for zero more than he did for any other score. And that is how batting is - failure is the norm.
Old television cricket broadcasts often showed a graphic breaking down the distribution of a batsman's scores - in which the lower scores were always more frequent. It is the crossing of this low-score barrier that often defines a successful innings, and it is batsmen who cross this barrier frequently enough who are labelled "consistent". This notion of consistency dominates discussions around the quality of batsmen.
Older analyses of consistency in batting have focused on measures like variance, which basically tells you how scattered the values in a set of data (in this case, a collection of scores) are. A low variance means more consistency in the data: many scores in the same range, and not too many highs and lows. However, batting scores are typically distributed in a skewed way: lower scores are far more frequent than high ones. In fact, the chances of making a score decrease sharply as the score increases: a player is ten times likelier to score ten runs than to score a hundred. The variance is not a good measure of spread for these distributions, because very high scores, which most batsmen make, can unfairly increase the spread.
To address the question of consistency, we go back to the more colloquial definition: does the batsman "succeed" often enough? Regardless of the academic definition of consistency, this is what we often imply when labelling batsmen consistent. Batsmen with long strings of failures are called inconsistent, while those with regular scoring patterns are not. Can we build a definition of batting consistency around success and failure and how they occur as a career progresses?
Our chosen idea of consistency relies on not only the number of successes but also their patterns of occurrence. Batting careers move through peaks and troughs, and a career with long troughs but high peaks is inconsistent in comparison with a career with a regular occurrence of good streaks, even though the two might have similar frequencies of "good scores".
How does one define a good streak? Our rule of thumb has two components: a) success, which we will define as a threshold score being crossed, and b) succeeding often enough, which we will define by setting a maximum number of failures between consecutive successes, which we call a "gap". These two parameters will together define what constitutes a "cluster" of successes, or a patch of consistency.
For example, let the threshold score for success be 25 runs, and let the "gap" be three innings. A cluster would be a set of scores where the batsman crosses 25 runs at least every third innings he plays. The cluster is broken when three or more failures occur between two successes.
How does one find these clusters in actual careers? Take a notional sequence of 15 scores, with a threshold of 25 runs and a gap of two innings. The scores are: 1, 34, 21, 32, 5, 7, 3, 8, 12, 123, 21, 56, 33, 4, 67. This is shown in the graphic below. The distance between the first and second success is one innings, which is less than two. Therefore, these two belong in one cluster. The distance between the second and third success is five innings, which is more than the chosen gap - these two do not belong to the same cluster. The third, fourth, fifth and sixth successful innings all lie close together to each other, as the distance between any two of them is fewer than two innings. All these together form another cluster. A mini-batting career is segregated into consistent clusters and voids of lean returns, by applying our colloquial definition of consistency.
Himanish Ganjoo/ESPNcricinfo Ltd
The relevant number to extract from this partitioning is the proportion of a career covered in these clusters. Batsmen with high averages will necessarily have a good proportion of high scores, but this measure reinterprets that in terms of frequency of success, or the regularity of scoring. Simply put, the proportion of a career covered in the clusters is the percentage of a career that a batsman has spent being consistent.
The numbers and sizes of clusters in a career are dependent on what threshold score and gap is chosen. We will consider two different definitions in this article: one where the threshold score is the batsman's median score, and one absolute definition that defines a successful innings as more than 50 runs.
To start, we will consider the median as a threshold for success, with one innings as the maximum gap. The median is a score that divides the batsman's career scores into halves: 50% of all innings are higher than the median, and 50% are lower. So, given that half of a batsman's knocks cross the median score, in an ideally regular career the batsman would cross the median score every second innings. In actual careers, streaks of high and low scores mean there are clusters of good performance. This isolates phases of extremely consistent scoring, as you are allowed only one failure between scores that exceed the median. We consider clusters that are four or more innings long, because we do not want to classify isolated islands of two high scores as clusters. We also consider not-outs as successes, simply because it is unfair to call them failures in any sense.
As an example, here is Steven Smith's career broken into such clusters. The blue shaded regions are clusters, while the black horizontal line denotes the batting average for that cluster. His career median is shown by the thin blue horizontal line, which forms the threshold. Of Smith's career as a whole, 59.7% is covered in these clusters, in which he has scored 73.9% of his total runs. In between, we see phases with high scores that are not clusters - these are phases with low scores punctuating a few very high scores that inflate the average. The cluster paradigm isolates phases that have a high average and consistent scoring.
Himanish Ganjoo/ESPNcricinfo Ltd
How does Virat Kohli fare on this paradigm? Compared to Smith, only 51% of his career is covered by such clusters, but he has scored 74% of his total runs in these phases. This confirms an often-debated feature of his batting: extreme highs broken by lean phases. Less of his career has been "consistent", but he has scored a larger amount of runs in those streaks compared to Smith.
Himanish Ganjoo/ESPNcricinfo Ltd
Don Bradman had 67.5% of his career in such clusters, which should set a (high) standard for this way of looking at batsmen, and bring some perspective to how often batsmen fail to score consistently. Only in two-thirds of his career could Bradman cross his median score once every two innings. Of course, this method uses a barrier that depends on the player in question. Bradman's median is higher than most other batsman's averages, so the threshold is very high in the first place. The figure for Jack Hobbs, 67.65%, is slightly higher than Bradman's, which puts Hobbs in first place.
Himanish Ganjoo/ESPNcricinfo Ltd
The thinking behind starting with a median threshold, rather than an absolute one, is to identify consistent batsmen who might not get chances to score in the top order. Ranking batsmen by the percentage of their career covered in these clusters, Adam Gilchrist and Rohan Kanhai are third and fourth respectively, both scoring 64.9%.
Himanish Ganjoo/ESPNcricinfo Ltd
With remarkably similar career lengths, they regularly exceeded their median returns. Gilchrist was the less volatile of the two, averaging 54.8 in clusters that had an average length of 14.8 innings, and 28.2 in other, non-cluster, innings. Kanhai had an average cluster length of just 7.4 innings, and averaged 60.56 in these clusters, falling to 23.9 in all other innings.
Himanish Ganjoo/ESPNcricinfo Ltd
Who are the others at the top? Considering all batsmen with 5000 or more Test runs, Cheteshwar Pujara has the highest proportion of career covered in clusters among currently active players. From the table, we see that the most consistent players spend 60% of more of their careers in these stringently defined streaks of performance, making 80% or more of their career runs.
ESPNcricinfo Ltd
At the other end, who are the lowest by percentage of career covered?
ESPNcricinfo Ltd
Just 40% of Kevin Pietersen's career was covered in clusters, while Kohli and Brian Lara, two heavy-scoring top-order batsmen with very high highs show up in the bottom 15. How do the cluster profiles of these batsmen look? We see narrow clusters that are often broken by stretches of inconsistent returns.
Himanish Ganjoo/ESPNcricinfo Ltd
Himanish Ganjoo/ESPNcricinfo Ltd
The above method of forming clusters is relative to a batsman's ability, and also extremely exacting, with the simple, tight condition of crossing your "middle" score almost every Test match. To contrast with this, we will now make clusters with an absolute definition of consistent scoring.
Crossing 50 runs, albeit an arbitrary number, is often considered a successful innings across conditions and situations. How many failures does one accept between two consecutive fifties? In a previous essay, I showed that elite batsmen have a gap of less than three to four innings between successive 50-plus scores on average. Constructing a new absolute definition of consistency, we can ask: For what proportion of their careers do batsmen have a gap of less than three innings between consecutive fifties? The rule for making clusters here: a gap of three or more innings between two fifties breaks the consistent streak.
Similar to the analysis above, we will look at how much of an individual career is covered in such clusters, considering clusters of five or more innings. These clusters correlate with high-average phases, but their definition is drafted in terms of succeeding often enough. How does Smith do on this metric? His career has six clusters, covering 88 innings out of his 139. He has scored 82% of his runs in these high-scoring clusters, which run for an average span of 14.67 innings each.
Himanish Ganjoo/ESPNcricinfo Ltd
Staying with the same example, we can glean three other important numbers from the construction of these clusters. First is the consistency factor, which is the ratio of the proportion of career innings covered in clusters to the proportion of career runs scored in clusters. A low consistency factor implies that the player has scored a high amount of his runs in a small proportion of his career: his highs have been very high and lows very low.
Secondly, this segmentation gives us information about exactly how good a batsman is during his high-scoring streaks, and how much he underperforms in his low-scoring streaks. To quantify this, we will look at the runs-per-innings of batsmen in clusters, and also the difference between run output in clusters and voids.
How do other top batsmen look in terms of clusters on the absolute metric?
Only 41.5% of Kohli's career falls in consistent clusters, and these account for 63.5% of his runs. His average cluster length is 12.2 innings, comparable to Smith's, but his average void length is much higher, at 14.33. Along with his low consistency factor of 0.65, this means that he has long stretches of indifferent returns between very high-scoring bursts.
Himanish Ganjoo/ESPNcricinfo Ltd
Sachin Tendulkar covered 54% of his 24-year long career in 13 consistent clusters, fairly evenly spaced, with an average cluster length of 13.7 innings. This includes a 34-innings long phase late in his career when he averaged close to 80.
Himanish Ganjoo/ESPNcricinfo Ltd
The table below gives the batsmen with the highest proportion of innings covered in clusters.
ESPNcricinfo Ltd
This table shows that top batsmen fulfill this consistency condition of scoring a fifty at least every third innings about 50-60% of the time over their careers. They score 70-80% of their runs in these purple patches.
The next table ranks the batsmen by consistency factor. A higher consistency factor means run-scoring was more equitably distributed throughout a batsman's career.
ESPNcricinfo Ltd
Joe Root* is among four elite players to have a consistency factor higher than 0.8.
Himanish Ganjoo/ESPNcricinfo Ltd
Another important question is how good batsmen are in their consistent patches, and how their performance varies with respect to lean patches. The next graphic shows the runs-per-innings in clusters plotted against their runs-per-innings in voids. Players who score more than 35 RPI are valuable because the propensity for scattered high scores ensures that they yield good average returns even when they do not score consistently.
Brendon McCullum, David Warner, Chris Gayle, Lara, Virender Sehwag, Younis Khan, and Root fall in this category. Players to the top right are high-output batsmen in fair and foul weather - their run-scoring is comparatively high even in lean patches, and their consistent clusters are extremely prolific.
The bottom-right segment contains Wally Hammond, Ken Barrington and Smith, with extremely bountiful clusters and poor voids. Their averages are high because big proportions of their careers are covered in clusters.
Himanish Ganjoo/ESPNcricinfo Ltd
With consistent performance defined clearly, we finally need two numbers to summarise a batsman's consistency. How much of their career is covered in these clusters? And, how good are they in these clusters? The graphic below shows this.
Himanish Ganjoo/ESPNcricinfo Ltd
Len Hutton, Hobbs, Smith, Kumar Sangakkara and Ken Barrington form an elite line on the top right, with high output in clusters, and a high proportion of careers spent being consistent. Sehwag, Kohli, Warner, Matthew Hayden, Pujara and Lara form another little group on the top left: they are heavy scorers when in hot streaks, but these consistent clusters cover less than 50% of their careers. Diagonally across, Root is a peculiarly consistent player, with around 55% of his career in clusters, but his cluster runs-per-innings figure is 54.67. Taken with the conclusions of the previous plot, Root is the least mercurial high-scoring player. His highs and lows are both reasonable, and he has been "consistent" for more than half his career.
*Feb 9, 7.15 GMT: Root is not the only current batsman on the list and the article has been amended to reflect that